Interoperable Knowledge Representation and Distillation for Integrated Process Planning in Hybrid Remanufacturing (Sponsor: NSF)
The overarching goal of this research is to establish a new framework of knowledge representation that leads to the concurrent optimization of hybrid manufacturing at the system integration level, which is facilitated by the cognitive principles producing unified and distilled knowledge. This framework bridges connectionist and symbolic approaches within knowledge graphs, systematically representing structured domain schemas and providing semantic richness to address data multi-modality, sparsity, and semantic heterogeneity in hybrid remanufacturing. The technical thrusts in this project are: (1) creating new cognitive encoders to unify multi-modal data from remanufacturing workflows into knowledge graphs, while integrating them as a retrievable memory into the semantic relationships; (2) advancing knowledge fusion through cognitive operations that facilitate data-driven reasoning, knowledge fusion, distillation, and online knowledge discovery; and (3) developing a symbiotic multi-objective optimization that leverages the memory-enabled knowledge graph to guide hybrid remanufacturing tasks. Collectively, these breakthroughs will be synergistically integrated into hybrid remanufacturing systems with insights from industry stakeholders.
Edge Computing in CNC Machining (Collaboration with CCAT)
While modern 5-axis CNC systems offer enhanced design flexibility and reduced production time, the dimensional accuracy of the workpiece is significantly compromised by geometric errors, thermal deformations, cutting forces, tool wear, and fixture-related factors. In-situ sensing, in conjunction with machine learning, has recently been implemented on edge devices to synchronously acquire and agilely analyze high-frequency and multifaceted data for the prediction of workpiece quality. However, limited edge computational resources and lack of interpretability in ML models obscure the understanding of key quality-influencing signals. This research introduces, a novel graph-based hyperdimensional computing framework that not only assesses workpiece quality in 5-axis CNC on edge, but also characterizes key signals vital for evaluating the quality from in-situ multichannel data. Specifically, a hierarchical graph structure is designed to represent the relationship between channels (e.g., spindle rotation, three linear axes movements, and the rotary A and C axes), parameters (e.g., torque, current, power, and tool speed), and the workpiece dimensional accuracy. Additionally, memory refinement, separability, and parameter significance are proposed to assess the interpretability of the framework.
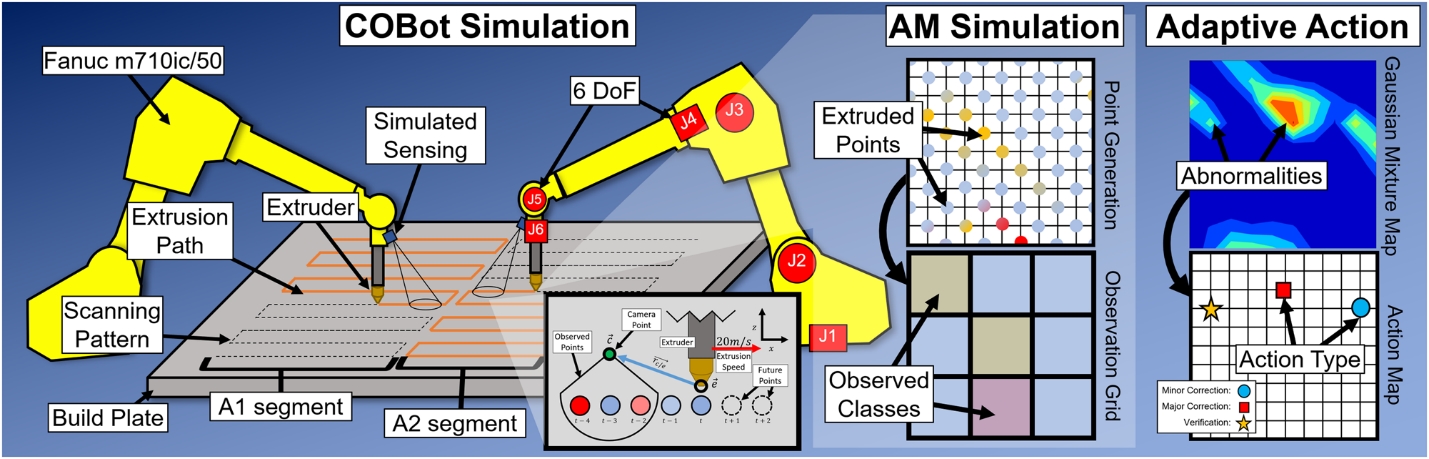